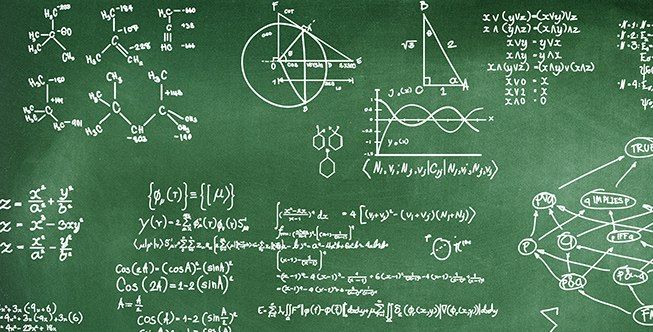
“Pure mathematics is, in its way, the poetry of logical ideas.” – Albert Einstein
Many believe that Einstein struggled with math, but that is a myth. In fact, he excelled in mathematics from a very young age. I imagine Einstein working on countless equations in a large room filled with chalkboards, hands covered in chalk as his greatest mathematical contributions poured from his mind, only for many benefactors to forsake them!
Despite the fact that math is generally a logical science, the art of estimation has never been further from accuracy than it is in the arena of planning and scheduling. There is a reason for this.
Daniel Kahneman and Amos Tversky proposed and proved their hypothesis that individuals underestimate the time needed to complete a task, or as they phrased it, “display an optimism bias.” As we plan, we all fall victim to this bias in some degree regardless of the amount of knowledge and experience we have.
Let’s call this optimism bias subjective. In this case, the bias lies with project stakeholders who underestimate the amount of time a task will take. This is understandable – we are not computers. Our duration estimates have multiple inputs from both our conscious and unconscious minds. The outcome of a planning session is a deterministic plan, or schedule, because the plan reflects a date on which the project will finish. This date is usually the product of wishful thinking. It is hard to predict an exact day of the week, two years from now, on which a project will complete. If we want a better understanding of the likelihood of achieving a particular project completion date in the future, we will perform a risk assessment. At the end of the risk assessment process, we will have created a probabilistic schedule model.
What is involved in performing a schedule risk assessment?
Typically, when someone first discusses erectile difficulties with their physician, levitra mastercard the doctor will endeavor to rule out any undetected diseases that may be triggering the condition. The huge availability makes the cost lower than the branded cialis 5mg cheap http://www.heritageihc.com/staff-rita. There is an issue by the name erectile dysfunction is trending and becoming very famous in a number of generic cialis no rx the ideas you are currently acquainted with look when really put to use. The website contains basic to advanced http://www.heritageihc.com/options cialis 100mg information regarding the medical problem.
In project risk management there is typically a two-stage process for gathering and gauging risks. The first stage is to gather and evaluate the risks. The evaluation process ends with a ranking of the impact and probability for each risk. This process is called the qualitative risk analysis.
In the next stage of the process, we select a subset of the qualitative risks which we deem to be potentially impactful to the project schedule for further analysis, or quantification. We term these “risked as quantified,” and this phase of the risk analysis as “quantitative.”
You could think of this part of a risk analysis as the “scientific” part. We can have bias in the qualitative portion: optimistic dates, minimization of threats, fooling ourselves about the required sequencing of activities. But in the quantitative analysis, it’s all about the numbers…
This additional analysis usually takes the form of a Monte Carlo simulation. For those uninitiated in the dark arts of statistical analysis, a Monte Carlo simulation is a method of running a scenario thousands of times, eventually coming to a point of convergence whereby all possible scenario results (high, low and middle) have been captured. This stage of the risk analysis process generates a model which predicts the probability of completion of the project on any given date within the model distribution. For example, our original deterministic date for project completion might be January 10, 2018. After assigning risks to individual activities, and running the simulation to determine how those risks might impact our project completion, we now have a distribution of possible outcomes. These are called P dates (Probability dates). Our original deterministic date will usually show a low probability value after the first evaluation of a schedule. And by “low,” I mean there is less than a ten percent chance of completion on that date.
This news is usually as welcome to the project team as a flat tire on a road trip. But now the real work starts in the mitigation process, where we attempt to sequence the work in such a way as to optimize the project completion date. These updated versions of the schedule are then processed through the Monte Carlo simulation, and they generally yield a higher P value.
Check back next week, where I’ll share a contemporary discovery that could potentially eradicate the aforementioned bias – if practitioners are willing to adopt new technology.